[Numpy] 분포함수(distribution)
- Python / Numpy
- 2021. 11. 20.
반응형
반응형
Numpy로 랜덤한 값을 기반으로 분포함수를 구현하는 방법입니다.
파이썬으로 하기 정말 간단한데 분포가 워낙 많이 있다보니깐 쓰는 함수만 쓰게 되긴 합니다만
복잡한 모델을 하다보면 평가방식을 달리 하거나 새로운 접근을 위해서 포스팅에 정리합니다.
구현
맛보기로 정규분포와 푸아송 분포를 구현해보겠습니다.
나머지 분포는 밑에 표로 남겨 놓겠습니다.
poisson 분포의 람다값은 1로 하겠습니다.
import numpy.random as npr
#샘플 사이즈
sample_size = 500
#분포
rd1 = npr.standard_normal(sample_size)
rd2 = npr.poisson(lam=1.0, size = sample_size)
# 그리기
from pylab import plt, mpl
plt.style.use('seaborn')
mpl.rcParams['font.family'] = 'serif'
%matplotlib inline
fig, (ax1,ax2) = plt.subplots(nrows=2, ncols=1, figsize=(10,8))
ax1.hist(rd1, bins=25)
ax1.set_title('standard normal')
ax2.hist(rd2,bins=25)
ax2.set_title('Poisson')
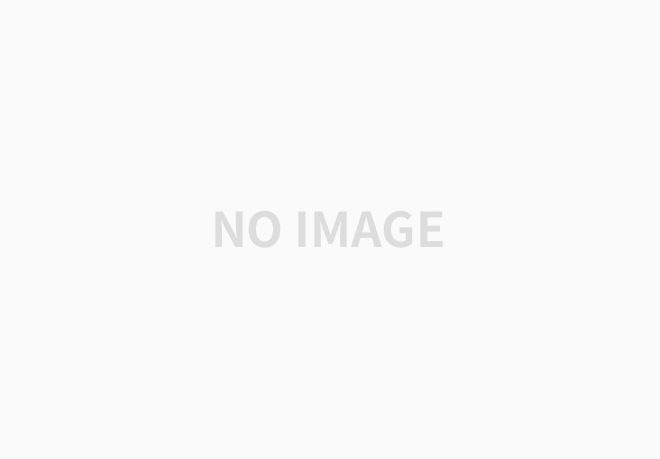
분포함수 정리
너무 많아 표로 정리합니다.
분포함수에 알맞는 파라미터에 적당한 값을 넣어서 구현하면 됩니다.
각 분포함수에 대한 정의나 성질은 추후에 포스팅을 하겠습니다.
함수 | 파라미터 | 출력 |
beta | a,b[,size] | beta distribution over [0,1] |
binomial | n,p[,size | binomial distribution |
chisquare | df[,size] | chi-square distribution |
dirichlet | alpha[,size] | Dirichlet distribution |
exponential | [scale,size] | Exponential distribution |
f | dfnum,dfden[,size] | F distribution |
gamma | shape[,scale,size] | gamma distribution |
gemetric | p[,size] | geometric distribution |
gumbel | [loc, scale, size] | Gumbel distribution |
hypergeometric | ngood,nbad,nsample[,size] | hypergeometric distribution |
laplace | [loc,scale,size] | Laplace or double exponential distribution |
logistic | [loc,scale,size] | logisitic distribution |
lognormal | [mean,sigma,size] | log-normal distribution |
logseries | p[,size] | logarithmic series distribution |
multinomial | n,pvals[,size] | multinomial distribution |
multivariate_normal | mean, cov[,size] | multivariate normal distribution |
negative_binomial | n,p[,size] | negative binomial distribution |
noncentral_chisquare | df,nonc[,size] | noncentral chi-square distribution |
noncentral_f | dfnum,dfden,nonc[,size] | noncentral F distribution |
normal | [loc,scale,size] | normal(Gaussian) distribution |
pareto | a[,size] | Pareto II or Lomax distribution with the specified shape |
poisson | [lam,size] | Poisson distribution |
power | a[,size] | power distribution with positive exponent a-1 in [0,1] |
rayleigh | [scale, size] | Rayleigh distribution |
standard_cauchy | [size] | standard Cauchy distirbution with mode=0 |
standard_exponential | [size] | standard exponential distrtibution |
standard_gamma | shape[size] | standard gamma distribution |
standard_normal | [size] | standard normal distirbution(mean=0,stdev=1) |
standard_t | df[,size] | Student's t distirubtion with df degrees of freedom |
triangular | left,mode,right[,size] | tirangular distribution over the interval[left, right] |
uniform | [low,high,size] | uniform distribution |
vomises | mu,kappa[,size] | von Mises distribution |
wald | mean, scale[,size] | Wald, or inverse Gaussian distribution |
weibull | a[,size] | Weibull distribution |
zipf | a[,size] | Zipf distribution |
관련 포스팅
[Python/Numpy] - ndarray 랜덤한값 생성
참고문헌
- Python for Finance
'Python > Numpy' 카테고리의 다른 글
[Numpy] 배열(array) 합치기(np.concatenate) (0) | 2022.01.05 |
---|---|
[Numpy] 배열 분할하기(np.split) (0) | 2022.01.03 |
[numpy] 행렬식, 고유값 계산하기(np.linalg) (0) | 2021.11.06 |
[Numpy] 소수점 반올림하기(np.set_printoptions) (0) | 2021.09.28 |
[Numpy]ndarray 저장, 불러오기 (0) | 2021.06.15 |
데이터목장님의
글이 좋았다면 응원을 보내주세요!